How is artificial intelligence used in fraud detection?
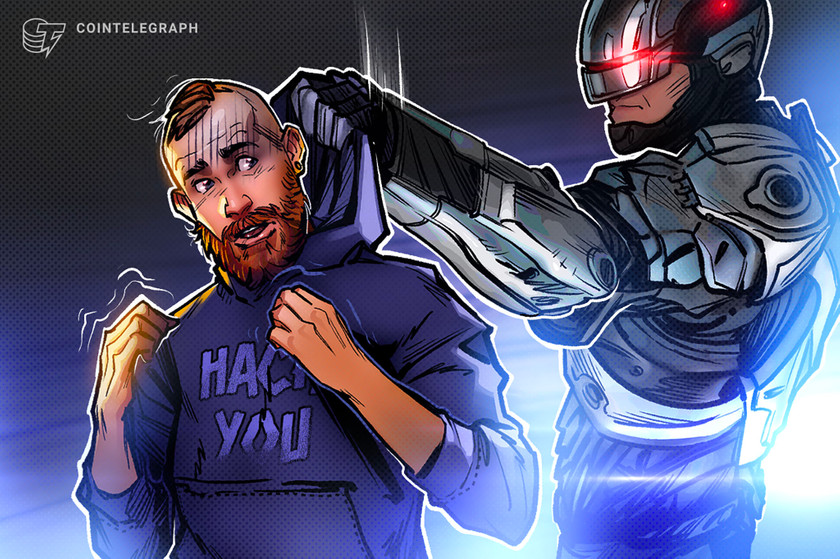
AI can contribute to fraud management by detecting data anomalies, identifying fraudulent behavior patterns and automating fraud detection processes.
What can AI do if a crime has already been committed?
The features of efficient data processing and pattern recognition can also be valuable features of AI in the case of forensic investigation.
Forensic investigation is the scientific method of researching criminal cases. It involves gathering and analyzing all sorts of case-related data and evidence. The nature of data is often complex, taking the form of texts, images or videos. AI can help handle data effectively and perform meta-analysis during the investigation.
AI algorithms can be trained to recognize patterns in data, such as handwriting, fingerprints or faces. They can be used to analyze written or spoken language, such as emails and text messages, as well as images and videos, to identify objects, people and events.
In addition, AI can aid in investigating and prosecuting the perpetrators. For instance, predictive modeling — a type of AI technology — can use historical crime data to create predictive models to help law enforcement anticipate and prevent future crimes.
To evaluate crime data and pinpoint regions that are more likely to experience criminal activity, police departments in some cities can use predictive policing algorithms. This enables them to allocate resources more skillfully and stop crime in its tracks. Predictive modeling can also be used to identify individuals at risk of committing crimes, allowing law enforcement to intervene before any criminal activity occurs.
What is the role of AI in crime prevention?
There are several existing solutions for crime prevention with the help of AI-based technologies; however, a few of them raise ethical concerns.
AI can be used in crime prevention by analyzing data that may indicate criminal activity. One example of an existing solution is the PredPol system, which uses machine learning algorithms to analyze historical crime data and identify patterns in the time and location of crimes. Based on these patterns, the system generates “predictive hotspots” that indicate where crimes are most likely to occur in the future.
A well-known example of fraud prevention in blockchain transactions is Chainalysis. The company applies machine learning algorithms to monitor and analyze the flow of cryptocurrency transactions across various blockchain networks. By analyzing the patterns of these transactions, experts can identify suspicious activities and track the flow of funds across different addresses and accounts.
The crime prevention system of China is a controversial example of AI-based solutions. The system relies on three pillars: Facial recognition tools help authorities to identify suspected criminals, big data tools allow police to analyze behavioral data to detect criminal activities, and a machine learning tool supports the creation of a database involving every citizen. The result is an extensive data-powered rating system that identifies suspicious individuals based on background and behavior signals.
It’s important to mention that AI in crime prevention has several limitations and raises serious ethical and privacy concerns. There are many debates about the accuracy and bias of some of these systems. It’s crucial to ensure they are designed and used responsibly, with proper safeguards to protect individual rights and prevent abuse.
How can criminals take advantage of AI?
The same features that make AI valuable for legitimate purposes can also make it a powerful tool for cybercriminals.
Here are a few examples of attacks that can happen if criminals exploit AI:
- Adversarial attacks: Adversarial attacks are a type of attack where fraudsters attempt to deceive or manipulate AI systems. For example, fraudsters may modify or manipulate data to evade detection or trick the algorithm into classifying fraudulent activity as legitimate.
- Malware: AI can be used to create and distribute malware designed to evade detection by security systems. Malware can be used to steal sensitive data, disrupt critical systems or launch attacks against other targets.
- Social engineering: AI can generate sophisticated phishing attacks designed to trick users into revealing sensitive information or installing malware on their devices. AI can also be used to create convincing fake identities and social media profiles, which can be used to deceive victims and gain access to their accounts.
- Botnets: AI can be applied to build and manage botnets, which are networks of infected devices that can be used to launch coordinated attacks against targets. Botnets can be used to launch distributed denial-of-service attacks and spread malware.
What are the potential risks of using AI in fraud detection?
Using AI-powered technologies also holds certain risk factors, which can be partly handled by explainable AI solutions.
The potential risks of AI in fraud detection are discussed below:
- Biased algorithms: AI algorithms depend on training data which can be biased. If the training data contains biases, the algorithm may produce inaccurate results.
- False positive or false negative results: Automated systems can lead to false positives or false negative cases. False positive means that a transaction is incorrectly labeled as malicious activity, while fraudulent activity is neglected in the case of false negative.
- Lack of transparency: Certain AI algorithms can be difficult to interpret, making it challenging to understand why a particular transaction was labeled as potentially fraudulent.
Explainable AI can help to partly overcome the incorporated risk factors. The term refers to the development of AI systems that can explain their decision-making processes in a way humans can understand. In the context of fraud detection, explainable AI can provide clear and interpretable explanations for why a particular transaction or activity was identified as potentially fraudulent.
For instance, The Montreal Declaration for Responsible Development of Artificial Intelligence outlines ethical principles for AI development, including transparency and explainability.
What are the main benefits of using AI in fraud detection?
Using AI in fraud detection can lead to a faster, more accurate and more efficient process without compromising the customer experience.
The key benefits are discussed below:
- Enhanced accuracy: AI algorithms can analyze vast amounts of data and identify patterns and anomalies that are difficult for humans to detect. AI algorithms can even learn from data and improve over time, increasing accuracy.
- Real-time monitoring: With AI algorithms, organizations can monitor real-time transactions, allowing for immediate detection and response to potential fraud attempts.
- Reduced false positives: One of the challenges of fraud detection is the occurrence of false positives, where legitimate transactions are mistakenly flagged as fraudulent. The learning feature of AI algorithms reduces false positives.
- Increased efficiency: AI algorithms can automate repetitive tasks, such as reviewing transactions or verifying identities, reducing the need for manual intervention.
- Cost reduction: fraudulent activities can have significant financial and reputational consequences for organizations. By reducing the number of fraudulent cases, AI algorithms can save organizations money and protect their reputation.
How can AI improve cybersecurity?
AI technologies have a vital role in fighting cybercrime by enhancing the most commonly used cybersecurity systems.
AI and ML have a crucial role in online fraud detection, where algorithms detect fraudulent activities in online transactions, such as credit cards, online banking or e-commerce transactions. These algorithms can be applied in real-time to identify and flag suspicious activities.
A cybersecurity threat is any activity, event or situation that has the potential to cause harm to computer systems, networks or data. According to the Global Economic Crime and Fraud Survey 2022, after customer fraud, the second most common type of threat that financial services face is cybercrime.
Cybercrime refers to criminal activities involving technology, such as computers, networks or the internet. These activities can result in various harms, including financial loss, data theft or destruction and reputation damage. The most common cyber threats include hacking, phishing, identity theft and malware.
A Cyberattack is a specific type of cybercrime that involves an intentional attempt by a third party to disrupt or gain unauthorized access to a system or network.
Cybersecurity is defending different systems, networks and devices from malicious attacks. A crucial element of cybersecurity systems is the real-time monitoring of all electronic resources. The biggest software companies, like IBM, already use AI-powered technologies to enhance their cybersecurity solutions.
How can machine learning algorithms help in fraud detection and prevention?
Machine learning algorithms are designed to recognize patterns based on a large amount of data, which can be leveraged to identify fraudulent activities.
AI refers to technologies that can perform tasks requiring human intelligence, such as analyzing data or understanding and responding to human language. They are designed to recognize patterns and make predictions in real time. AI algorithms are often a combination of different ML models.
ML is a subset of AI; it uses algorithms to analyze large amounts of data to enable systems to learn autonomously. The more data ML algorithms are exposed to, the better they perform over time. The two main approaches of ML are supervised machine learning (SML) and unsupervised machine learning (UML). SML algorithms use labeled data to help predict outcomes, while UML algorithms discover hidden patterns in the data.
As an example, SML algorithms use historical transaction data labeled as fraudulent or non-fraudulent that will be used to train the supervised machine learning model. UML would use anomaly detection algorithms to identify transactions significantly different from the norm based on given features. While UML models require less human intervention, they tend to be less accurate than SML.
How can artificial intelligence help detect fraud?
Artificial Intelligence can play a crucial role in fraud management by detecting and preventing fraudulent activities.
The global average rate of losses caused by fraud for the last two decades represents 6.05% of the gross domestic product. Additionally, companies have reported that cyber breaches have caused financial damages equaling 3% to 10% of their revenue. Moreover, global digital fraud losses are projected to exceed $343 billion between 2023 and 2027.
Given the estimated amounts, it is a crucial question for any organization to build up an efficient fraud management system. Fraud management is identifying, preventing, detecting and responding to fraudulent activities within an organization.
Artificial intelligence (AI) has a significant role in fraud management. AI technologies, such as machine learning (ML) algorithms, can analyze large amounts of data and detect patterns and anomalies that may indicate fraudulent activities. AI-powered fraud management systems can identify and prevent various types of fraud, such as payment fraud, identity theft or phishing attacks. They can also adapt and learn from new fraud patterns and trends, improving their detection over time.
AI-based solutions can also integrate with other security systems, such as identity verification and biometric authentication, to provide a more comprehensive approach to fraud prevention.